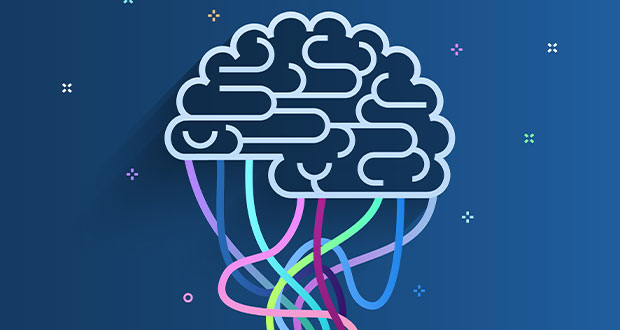
DEPOSIT PHOTOS
Part of the “magic” of generative AI is that one can put in a request and get an answer without doing the grunt work of parsing through mountains of data or manually incorporating visual elements in a creative process – it is a shortcut to a final product.
But part of that magic is that one cannot see behind the curtain. Most AI users don’t know where the AI they are using “keeps its brain” and part of that is that they do not know what data are being utilized to generate the answer, the AI’s process to move from these data to the answer, nor what data were used to teach the AI how to arrive at the answer. The veracity of an answer produced by generative AI is wholly dependent on data its algorithms access, not just to produce the answer, but also, the data that (continually) trains its algorithms.
Much generative AI is continuously self-learning so maintaining data quality is an ongoing issue. Database programmers are all familiar with the old adage: “Garbage in, garbage out.” The data integrity challenge is not new, but with AI, the necessity is that the data remain constant through the life and use of the AI. The importance of this is paramount especially because one cannot really see where it “keeps its brain” so seeing what is shaping this brain is important.
Data utilized by AI algorithms is not a neat and tidy proposition. If one is utilizing AI with a closed universe of data from which to produce answers, a user can arguably feel more secure that the results are not hallucinations (false information created/supplied by AI). But receiving an answer that is not a hallucination is far different than receiving a best answer. One can close and carefully curate data drawn upon for answers (understanding the risks of stale data being provided to form the answer), but one cannot as easily limit the data the AI uses to train itself to provide those answers.
In an ideal world, where every user who interacts with AI is attempting to follow best practices, the AI would learn and improve and become more useful in responding to requests; but not all users are good actors and, whether because they are nefarious or just bored, some users may take great enjoyment from interacting with AI and through these interactions, training it to “think” differently.
Controlling AI interactions to avoid incorrect answers or interactions, whether they are hallucinations or just offensive, can adversely affect the AI’s functionality as well. When chatbot Tay lasted only 16 hours on social media before its posts became wildly offensive, Microsoft replaced it with Zo. Zo had fewer instances of making offensive posts, but to address these posts, Zo was prevented from chatting about various subjects. Zo’s functionality was compromised and Zo was accused by investigative journalist Chloe Rose Stuart-Ulin of being “a judgmental little brat” when one attempts to converse with it on forbidden subjects.
To avoid hallucinations, best practices include data transparency. In this case, data transparency means understanding not only the data from which AI will pull results but understanding what data is and can be utilized to train the AI. An advantage of machine learning used by AI is speed. Machine learning algorithms can digest significant amounts of data and find patterns and relationships both to train the algorithms and to provide answers that could not reasonably be provided by a human or a team of humans within a workable amount of time.
But there is a risk in not knowing the content and integrity of the data. One could argue that the necessity to monitor the data compromises the speed at which the AI can provide results; but understanding the data is how one can see (to any degree) the brain of the AI.
When receiving an answer from an AI engine, one may wish to ask not only whether the answer is correct, but also, the practices surrounding the data that formed the foundation of the answer. Proprietary creators of AI are arguably less likely to share the details of the algorithms, but the practices surrounding maintaining the integrity of the data that the AI utilizes, both to learn and to respond, are useful avenues of inquiry.
Rachel L. Pearlman is a partner in the Albany office of Heslin Rothenberg Farley & Mesiti P.C.